How AI Can Reduce Participant Bias
The path to honest consumer insights can be long and winding. Removing any obstacles in your way is key if you want to uncover the truth through research.
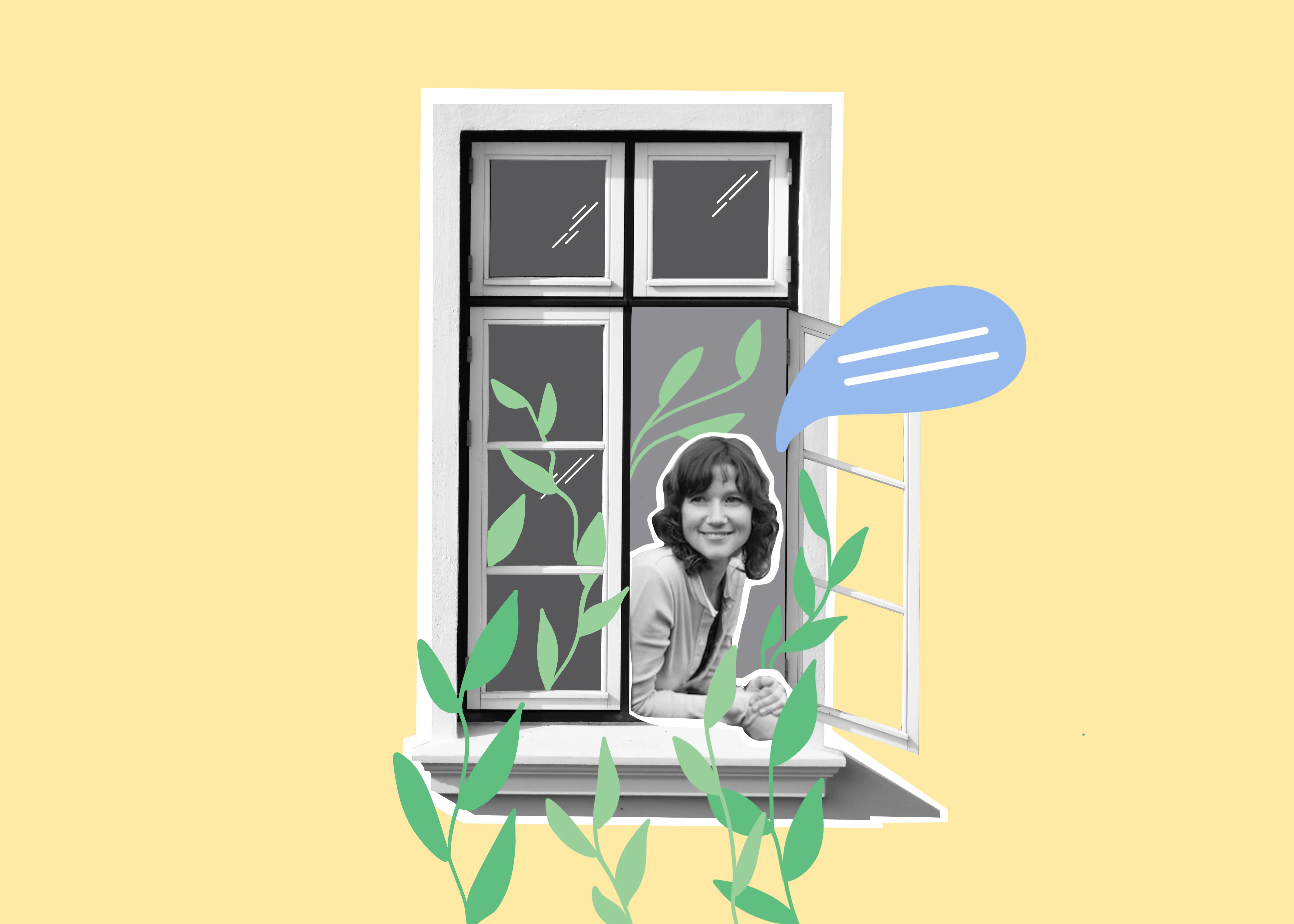
The path to honest consumer insights can be long and winding. Removing any obstacles in your way is key if you want to uncover the truth through research.
One of the biggest barriers quantitative and qualitative researchers face is bias. This is especially true in qualitative research, where bias can be difficult to reduce or prevent.
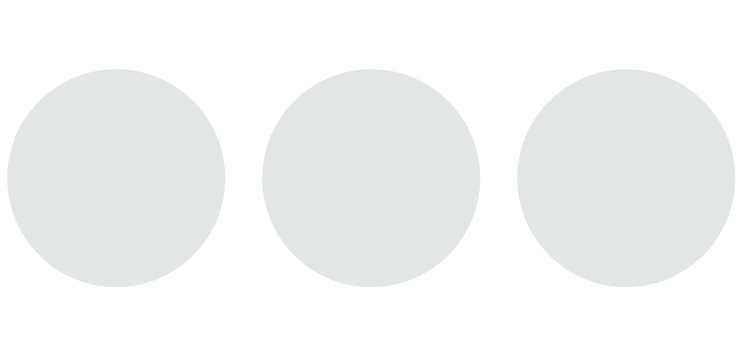
What is Participant Bias?
Bias happens when an outside factor influences participant response in a way that results in prejudiced results. Participant bias occurs when a participant provides a response that s/he thinks is correct, accepted, or appropriate, rather than responding in a manner that reflects honest opinions or reports actual behaviors. This type of bias can be a major barrier to uncovering truth.
Artificial intelligence (AI) and natural language processing (NLP) are two emerging innovations that can help researchers decrease participant bias. Here are seven ways participant bias can show up in research, and seven ways AI and NLP can reduce bias.
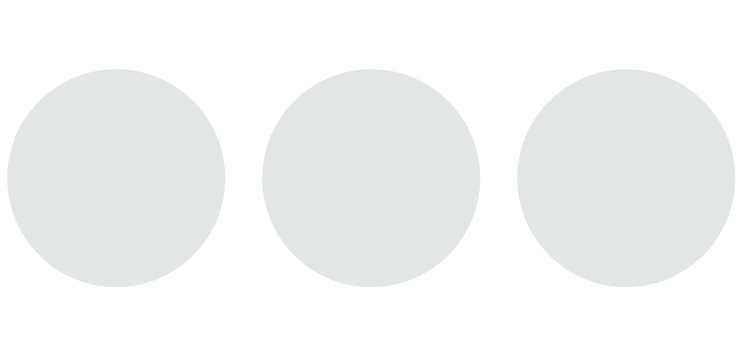
7 Types of Participant Bias Problems...
And How AI Can Help Solve Them
There are many factors that can influence participant bias and errors in research. The first step to reducing bias is learning more about the most common types of biases. Here are some of the ways participant bias may occur in your research and how AI and NLP can offer solutions.

The Problem: Response Bias
Response bias is when a participant answers questions in a way that is false or inaccurate. This can happen for many reasons, and it results in research errors.
Sometimes a participant does not realize they are answering falsely, which results in unconscious bias. Response bias can also be conscious, as a result of the way participants perceive the researchers or the study. Both types of responses bias hurt research and cause research error.
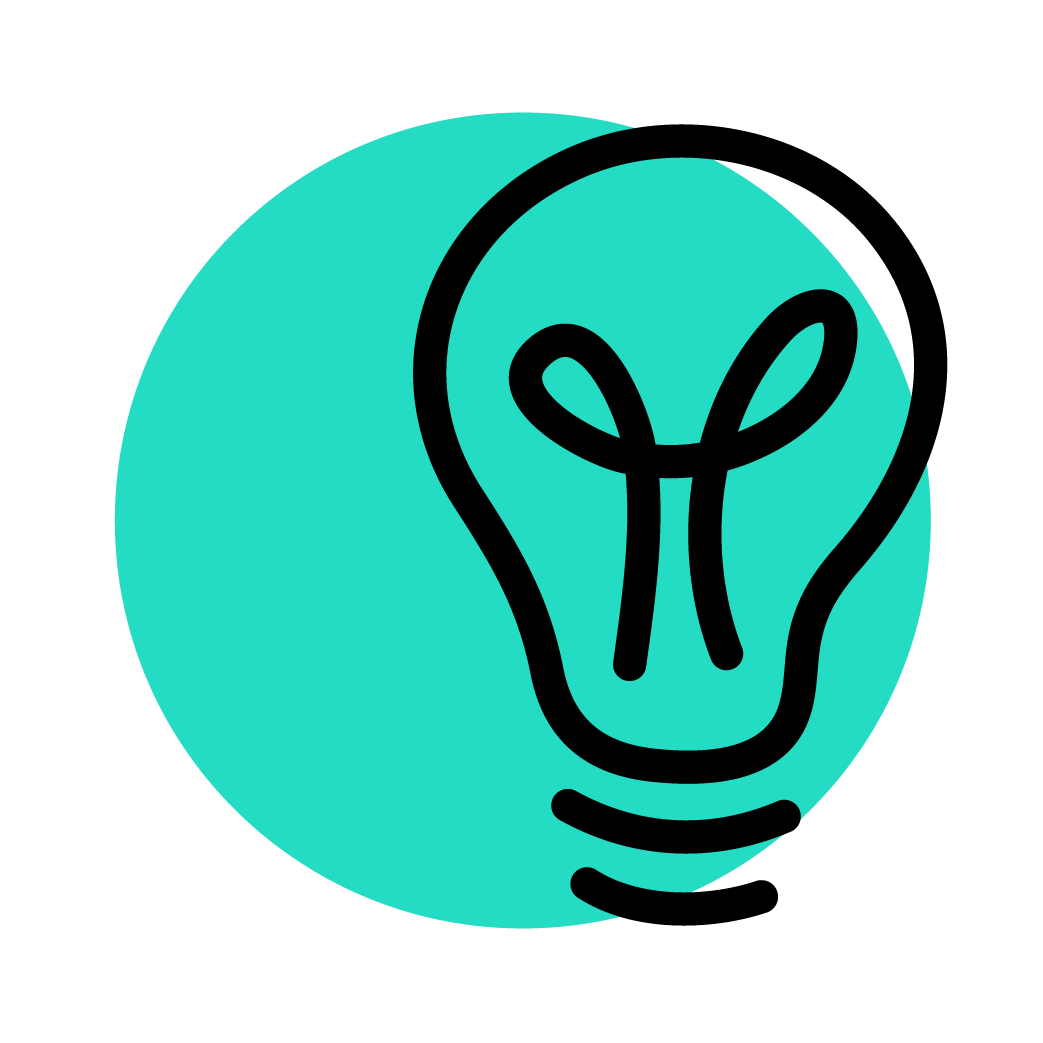
The Solution: Researcher Anonymity
Demand characteristics, social desirability, and conformity bias can all result from the way a participant sees a researcher. With AI, research can be conducted and evaluated digitally, reducing researcher and participant facetime and reducing potential researcher influenced bias.
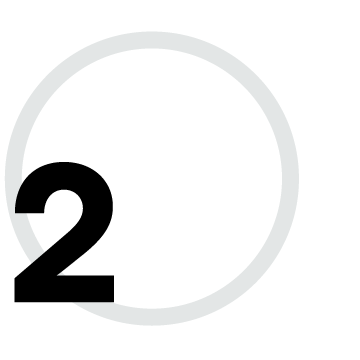
The Problem: Social Desirability and Conformity Bias
One of the most common reasons a respondent may not answer questions truthfully is due to social desirability and conformity bias. When a participant feels they might be judged for a response they are less likely to be honest with their answers. In fact, research suggests that this type of bias may result in 10% to 75% of variance in participant responses.
Public opinion matters a great deal to participants. Particularly in studies that require people to self-report on behaviors or opinions that have moral weight, participants will change their answers to make themselves look better. Participants will also sometimes change their answer to fit in with the group. This can present a real problem for researchers who are searching for the unbiased truth.
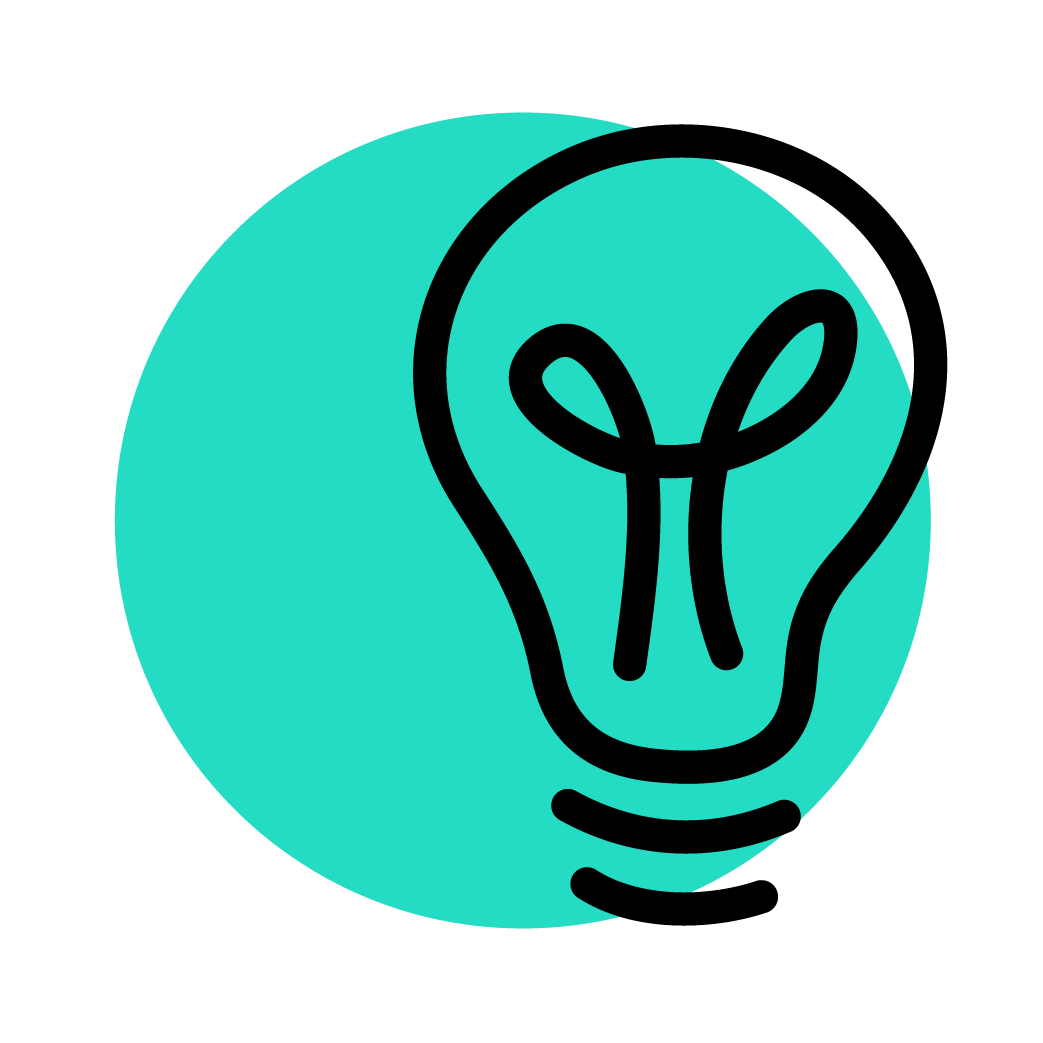
The Solution: Participant Anonymity
For many participants, the idea of judgement from other participants or researchers can influence their answers. One benefit of AI and NLP research methodologies is that participants can remain anonymous. This significantly reduces the likelihood of social desirability and conformity bias.
On platforms like Remesh, participants are also prevented from seeing other participant responses before offering their own. This ensures that other participants can’t influence opinions and feedback.
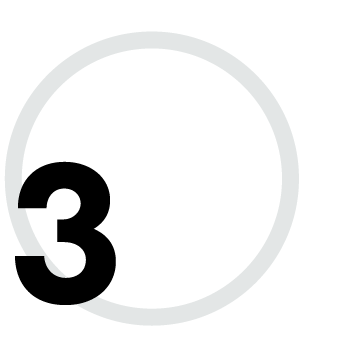
The Problem: Acquiesce and Agreement Bias
Sometimes participants are inclined to agree with statements that are positive or that have a positive connotation. Some participants want to appear positive and agreeable, and some are influenced by positive reinforcement from researchers. However, this can often result in an inaccurate positive research result that does not truly reflect consumer opinions.
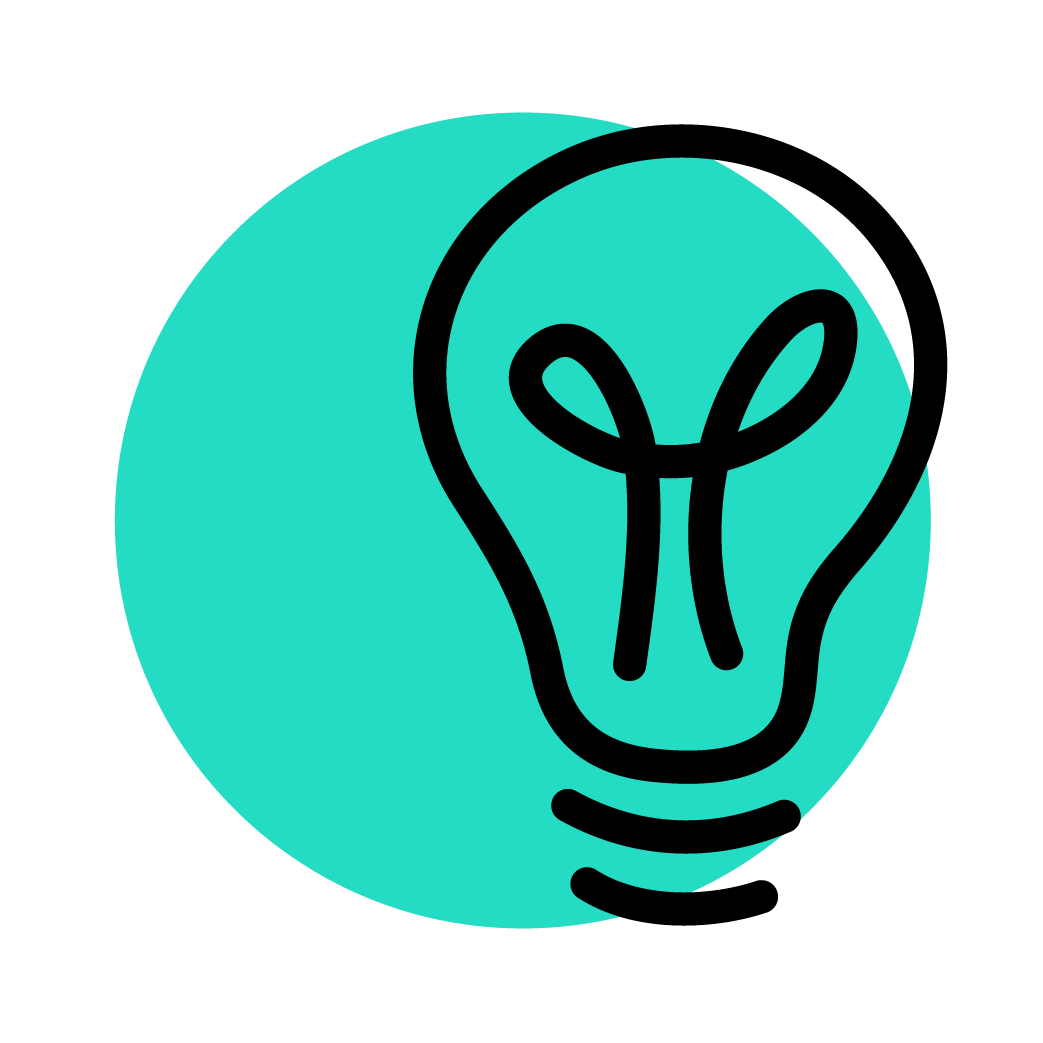
The Solution: Authentic Consensus Data
In some traditional research methodologies, one participant's voice can dominate the conversation, influencing other participants. Utilizing AI, researchers can ensure that the most popular responses, not just the loudest, rise to the top. When participants blindly vote on other participants’ feedback, they help the AI determine the popularity of each response.
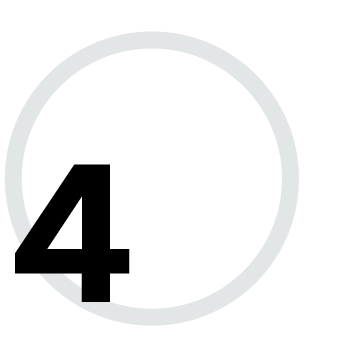
The Problem: Central Tendency Bias
Some respondents do not want to answer questions in any extreme direction. As a result, they will stick to answers that are mild or middle of the road. This is called central tendency bias.
This occurs most often in quantitative research where participants are responding using metrics like a Likert Scale or NPS score. However, this can also occur in qualitative research. A participant’s desire to appear more neutral than they really are can result in data that is skewed towards neutrality.
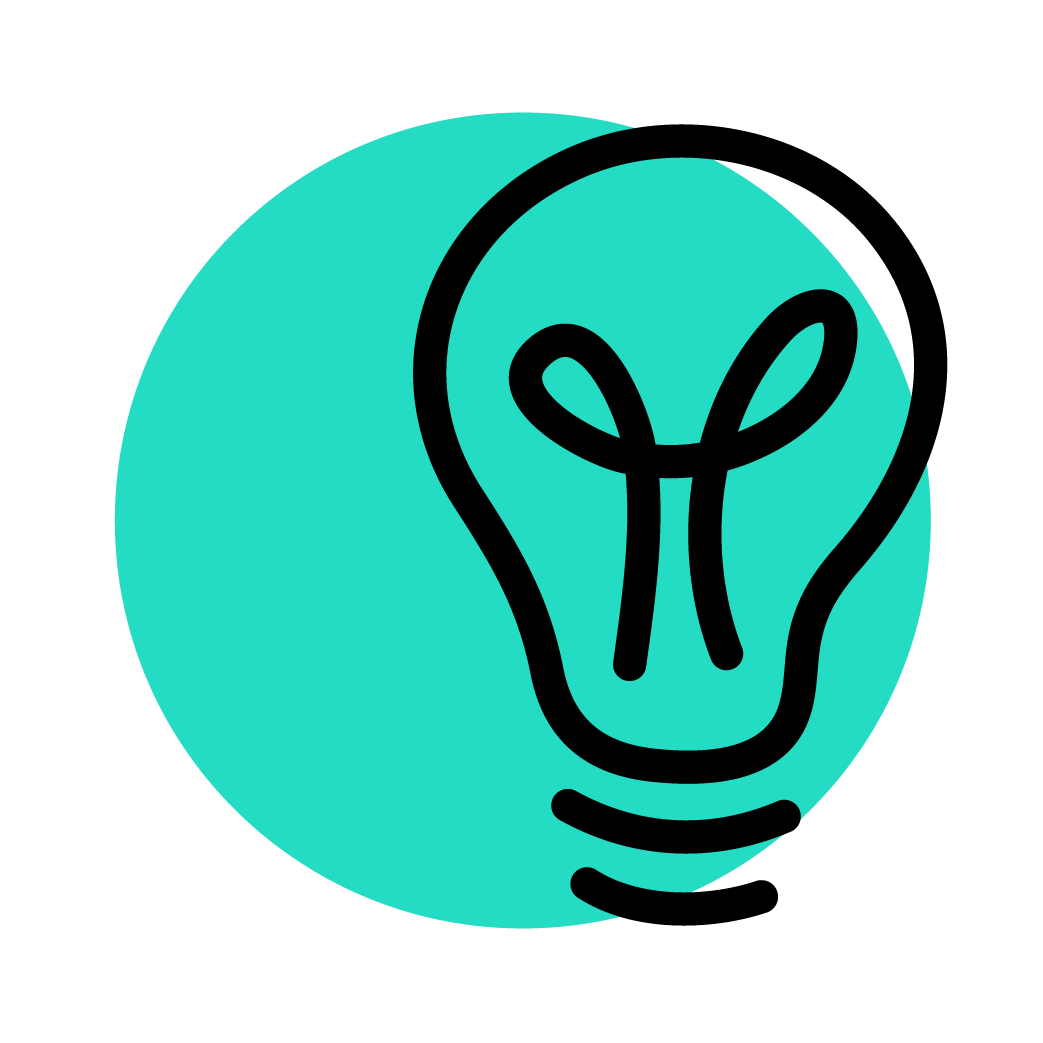
The Solution: Open-ended Feedback, At Scale
Central tendency and agreement bias can be difficult to combat, especially when researchers are looking for at scale data. With most traditional methodologies, you need to choose between open-ended questions and a larger sample size. Or, you must conduct separate qualitative research to check quantitative results.
AI and NLP give researchers the best of both worlds. The speed of data analysis that AI enables means that participants can provide open-ended feedback in addition to quantitative data. The combination of these two responses can deliver more honest feedback from participants, resulting in less central tendency and agreement bias.
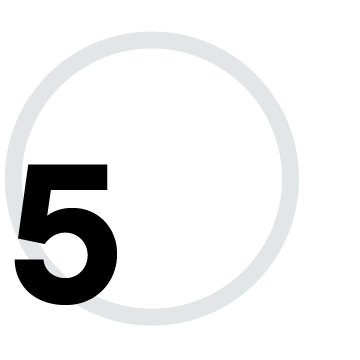
The Problem: Demand Characteristics
When a participant senses a cue from a researcher on expected findings, this can influence their answers. Sometimes this type of bias makes a participant answer in the way they know they are expected to. Sometimes a cue from a researcher makes them disagree with the expected responses. Either way, demand characteristics often result in false research results.
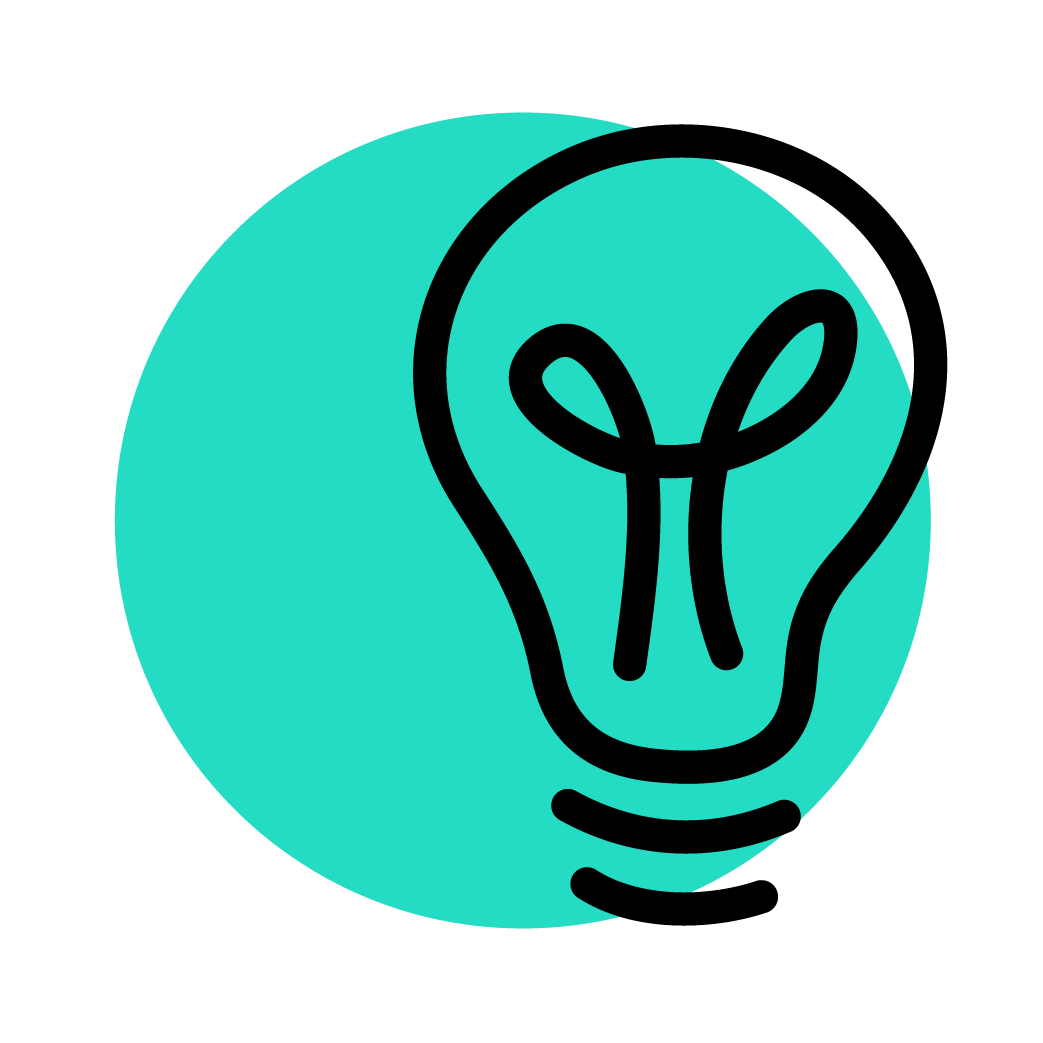
The Solution: Limited Moderator Influence
AI and NLP technology adds an additional barrier between participants and researchers. In a virtual setting, AI can help prevent confirmation bias from occurring. This is because participants cannot see or hear researchers' reactions to their answers. Additionally, participants cannot pick up on non-verbal cues or body language that may impact their answers.

The Problem: Habituation Bias
Habituation bias happens when participants give similar or identical responses to similarly-worded or related questions. This often occurs because of survey fatigue or because participants are bored or confused.
In quantitative research, this type of bias can result in respondents choosing the same answer repeatedly. In qualitative research, participants may offer similar opinions repeatedly. In both cases, the data being collected is not representative of the participants' true feelings.
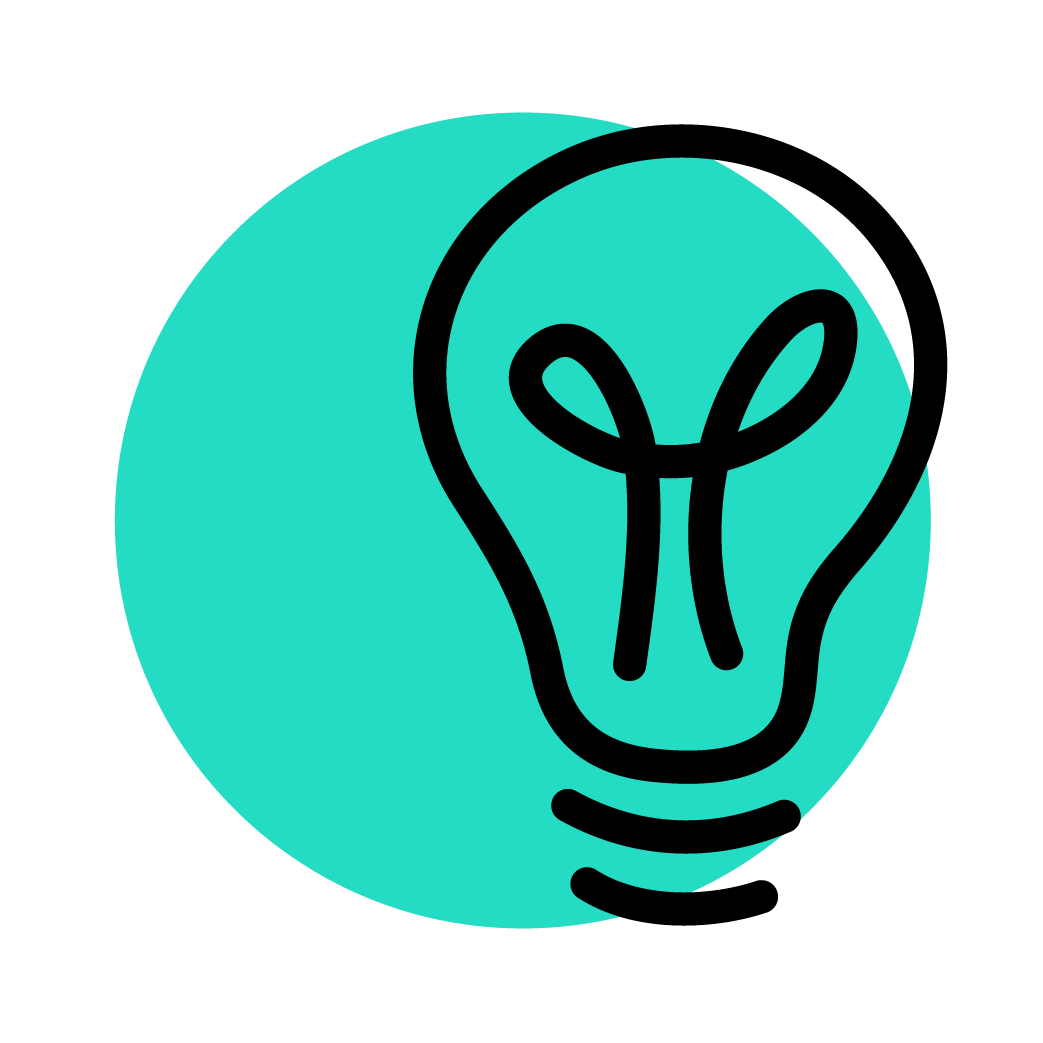
The Solution: Conversational Agility
Sometimes even the best-laid plans of researchers cannot predict when participants will give the same answer to several different questions. In traditional qualitative and quantitative research, it can be difficult and time consuming to add additional questions during the study.
Research methodologies that use AI are often efficient and agile. This gives researchers the ability to add questions on-the-fly. This means that when participant responses are similar to two questions, researchers can quickly add new questions to the study. This can help to clarify responses or seek a different response. These added questions can also be used to break up a study and address survey fatigue and boredom.
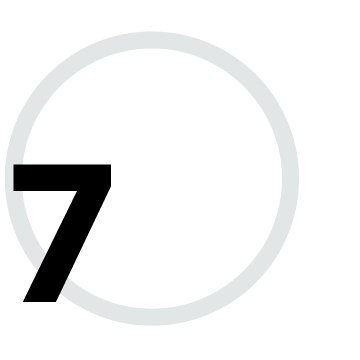
The Problem: Satisficing
While every researcher hopes that participants will take a study seriously, this does not always happen. Satisficing is when a participant only completes the bare minimum required of them. In a quantitative study this may result in skipping questions or speeding through questions. In qualitative research satisficing may also result in skipped questions or short and incomplete answers.
Satisficing can skew research results, especially when the sample size is low. There are no guaranteed ways to force participants to take questions seriously. However, there are ways to help account for this type of bias.
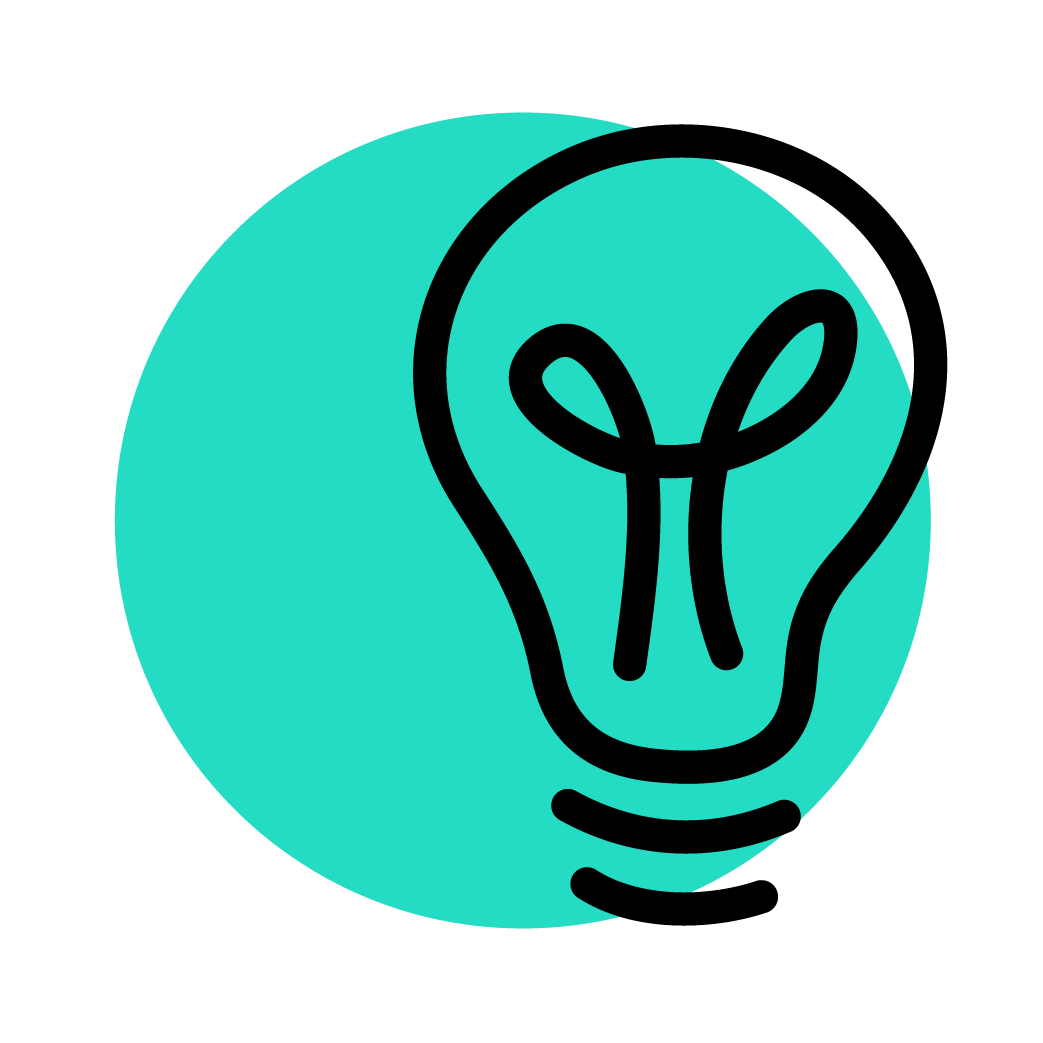
The Solution: Crowd-Sourced Consensus Data
As previously mentioned, AI and NLP research methodologies can provide opportunities for participants to rank other participant responses. These technologies can also enable increased sample sizes. Both of these capabilities help researchers weed out participant responses that are a result of satisficing.
Participants will not vote for responses that are poor quality, and the best answers will rise to the top. An increased sample size will yield more accurate results and have less satisficing answers to skew results.
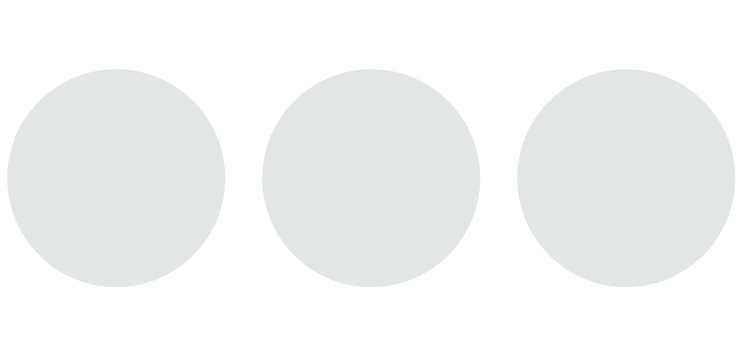
Case Studies on Reducing Participant Bias Using AI
Looking for some inspiration on how AI can help you reduce participant bias? Check out some of the case studies below.
- Employee Engagement – Egon Zehnder utilized Remesh to help a global client uncover key diversity & inclusion issues. The platform anonymity increased participant honesty and uncovered actionable insights.
- Purchasing Habits – A shampoo brand leveraged Remesh to capture scalable insights about several new product concepts. The mix of quantitative and qualitative feedback gave the brand concept validation and insights into the “why” behind participant responses.
- Loyalty Program Concept Testing – An international fast food brand needed to capture honest consumer feedback on loyalty program concepts. The Remesh platform’s conversational agility enabled the team to add on-the-fly questions as unexpected insights arose.
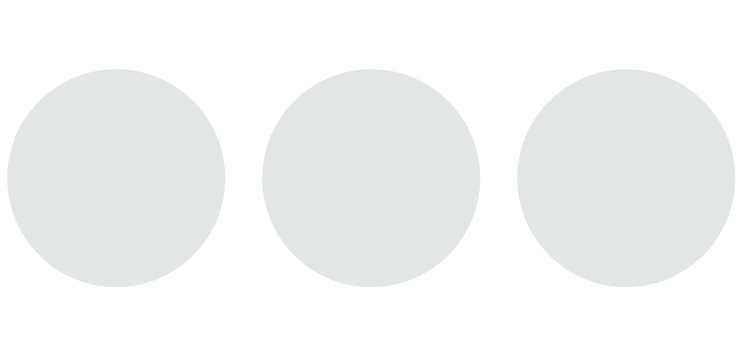
Start Reducing Participant Bias
There are many tools and recent innovations in the insights industry that can help reduce participant bias. AI and NLP methodologies are rapidly becoming go-to solutions to reduce participant bias.
Participant bias can be difficult to address, and it can damage the truth of your research results. Stay ahead of the industry, and use these tools to your organization's advantage. {{cta('4924012b-eaba-41b9-87de-f1de423074bb')}}
More
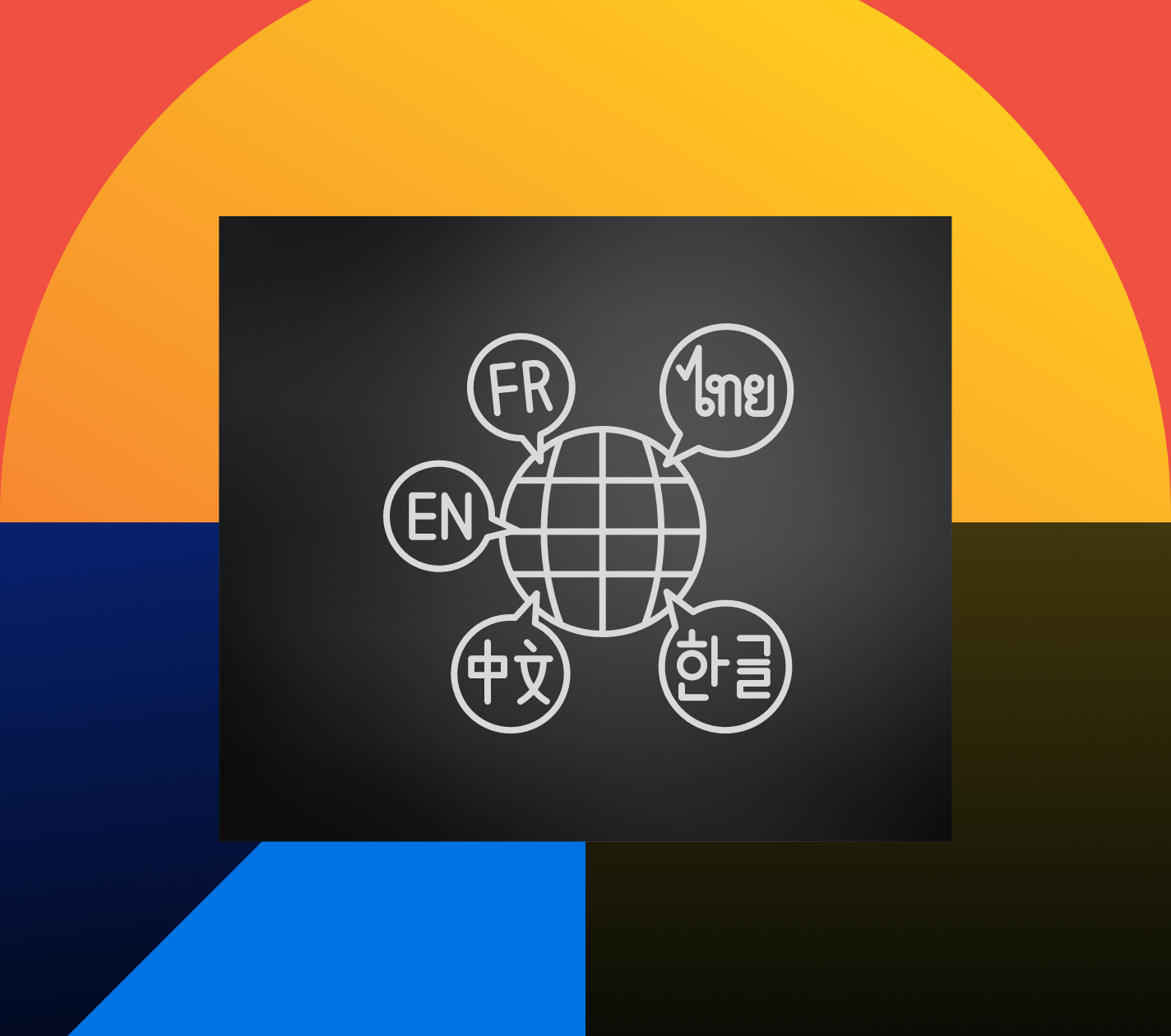
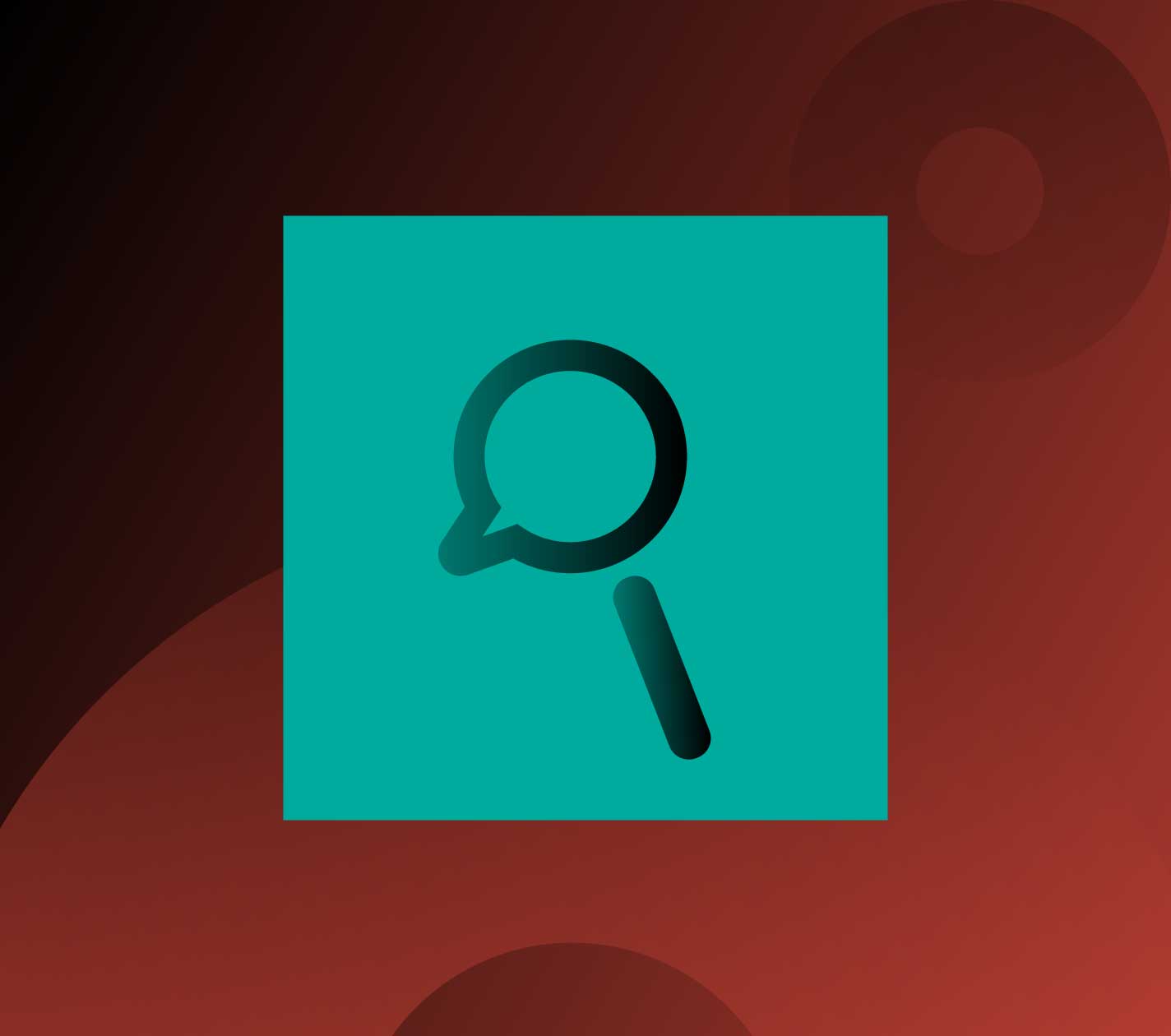
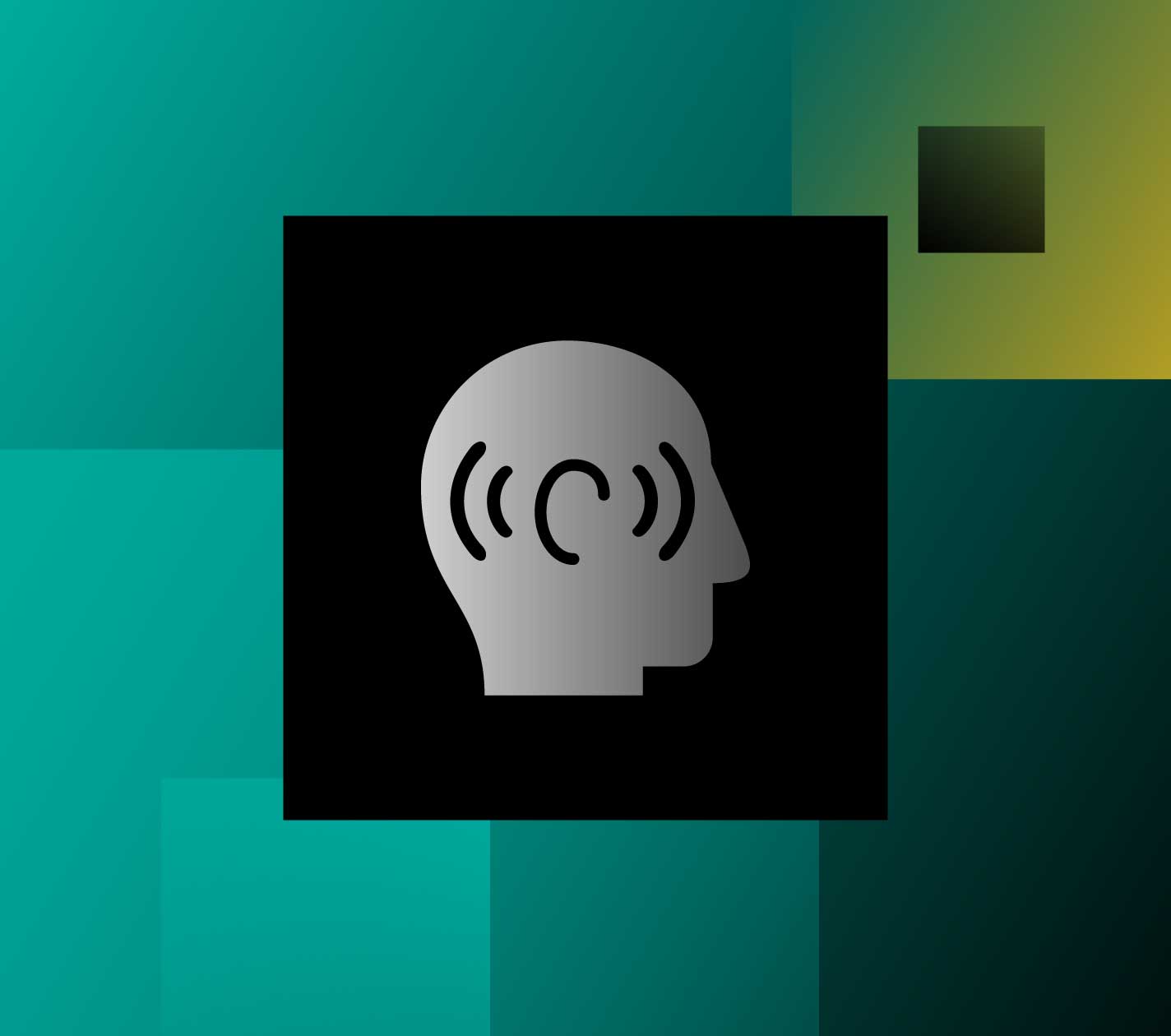
Stay up-to date.
Stay ahead of the curve. Get it all. Or get what suits you. Our 101 material is great if you’re used to working with an agency. Are you a seasoned pro? Sign up to receive just our advanced materials.