.jpg)
AI
The Modern Researcher’s Guide to AI Analysis Tools
Jessica Dubin
February 19, 2025
Articles
.jpg)
AI
The Modern Researcher’s Guide to AI Analysis Tools
Jessica Dubin
February 19, 2025
Articles
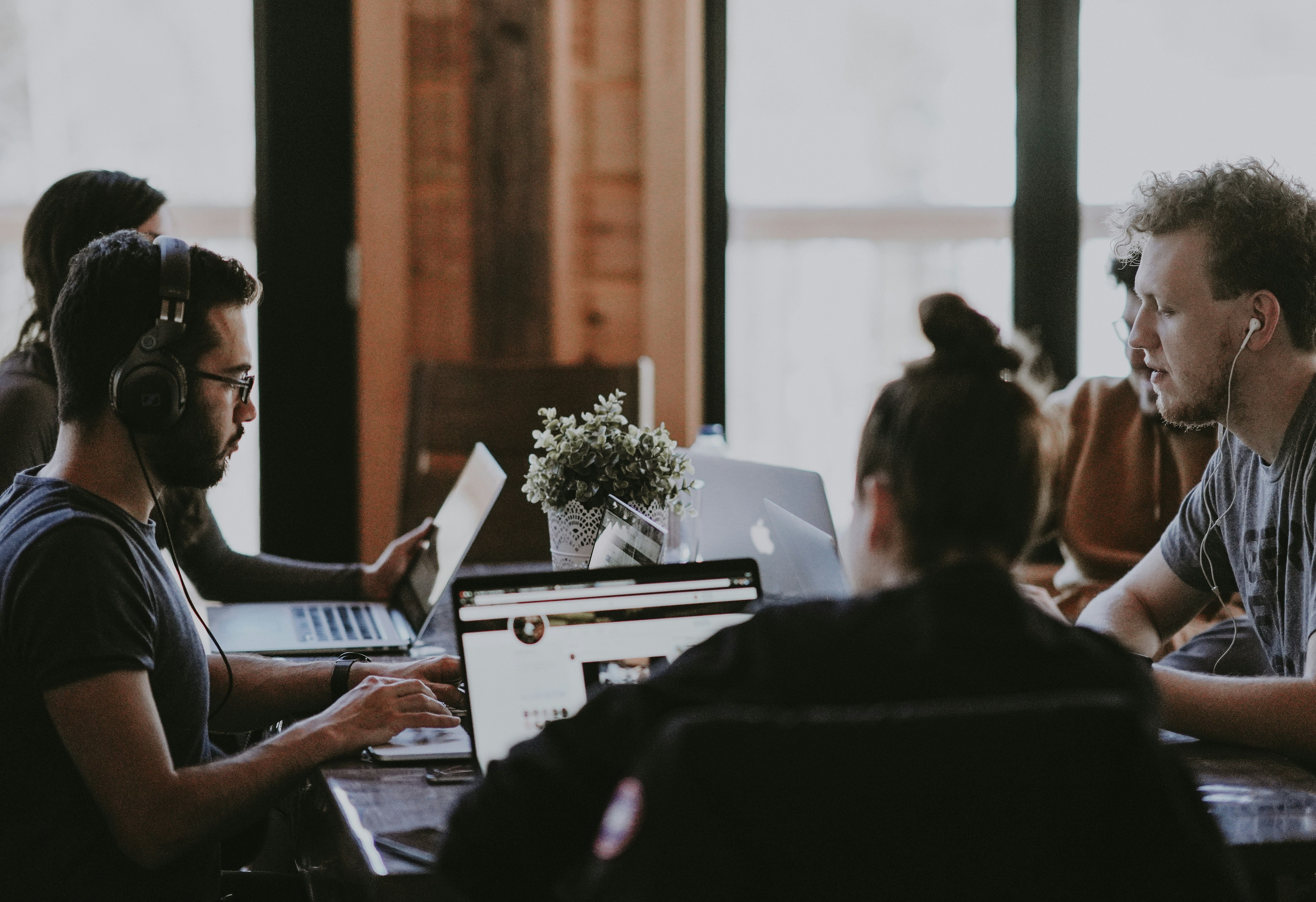
Advanced Research
Workplace Realities in 2025: What Employees Are Saying
Patrick Hyland
February 10, 2025
Employee Research
Articles
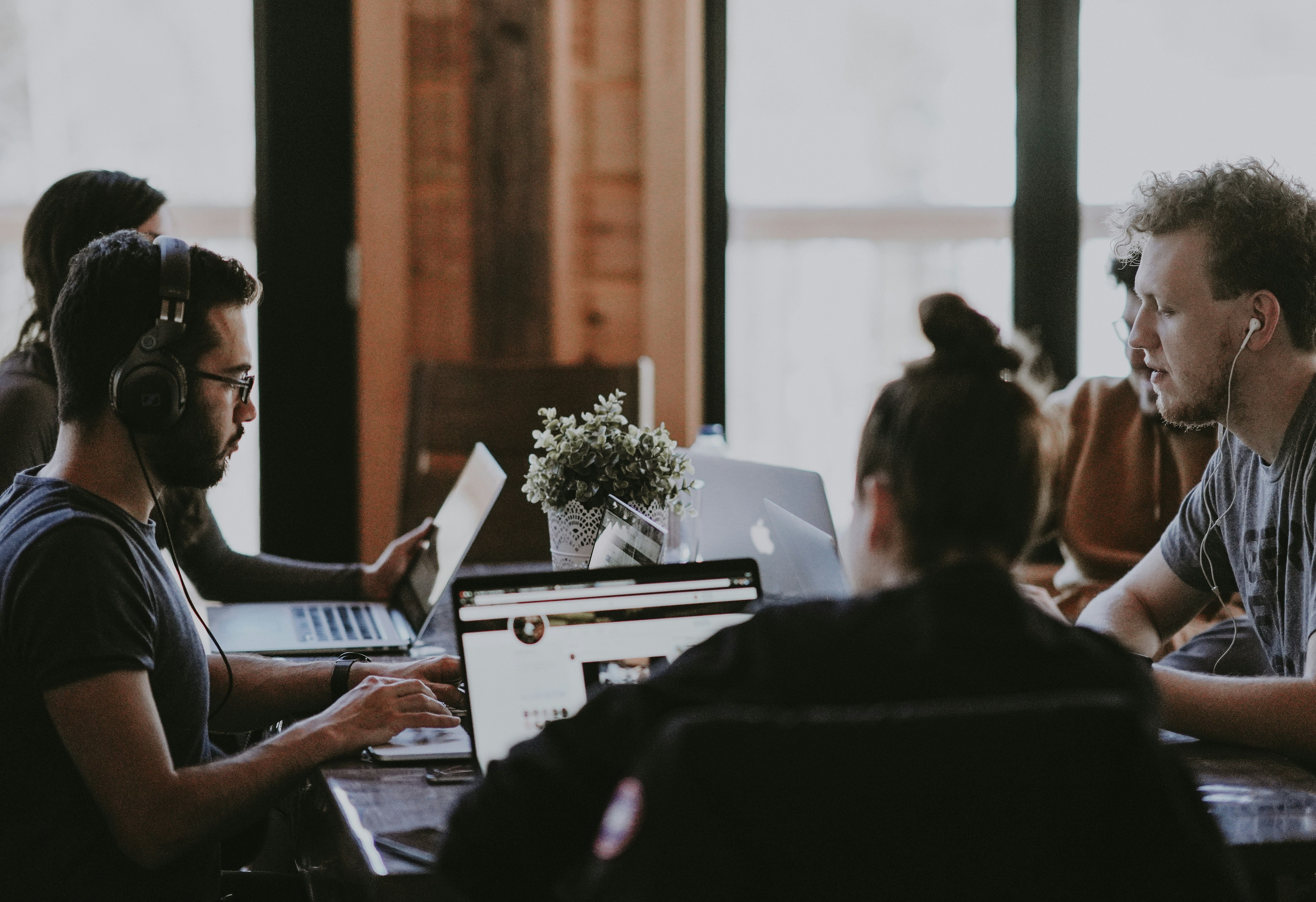
Advanced Research
Workplace Realities in 2025: What Employees Are Saying
Patrick Hyland
February 10, 2025
Employee Research
Articles

Advanced Research
Taking a Dialogic Approach to Change
Patrick Hyland
January 10, 2025

Advanced Research
Taking a Dialogic Approach to Change
Patrick Hyland
January 10, 2025
.jpg)
Advanced Research
Creating a Culture of Belonging: Insights Every Leader Should Know
Patrick Hyland
January 10, 2025
Articles
.jpg)
Advanced Research
Creating a Culture of Belonging: Insights Every Leader Should Know
Patrick Hyland
January 10, 2025
Articles

Research 101
Ideation Tool: How Remesh Transforms Idea Collection and Testing
Team Remesh
November 12, 2024
Market Research
Articles

Research 101
Ideation Tool: How Remesh Transforms Idea Collection and Testing
Team Remesh
November 12, 2024
Market Research
Articles

Advanced Research
How Gen Z Leadership Expectations Are Shaking Up the C-Suite
Anthony Caputo
November 4, 2024
Articles

Advanced Research
How Gen Z Leadership Expectations Are Shaking Up the C-Suite
Anthony Caputo
November 4, 2024
Articles

Advanced Research
Qualitative Research Methods: When Medium Matters (And When It Doesn't)
Team Remesh
November 4, 2024
Articles

Advanced Research
Qualitative Research Methods: When Medium Matters (And When It Doesn't)
Team Remesh
November 4, 2024
Articles
How Large Language Models (LLMs) are Shaping the Research Industry: Benefits, Limitations, and Risks
Remesh CEO Gary Ellis and CTO Dan Reich shed light on how Remesh is using LLMs, and what researchers need to know as they navigate this ever-changing landscape.

Generative AI is disrupting every industry and the Research industry is no exception. In particular, Large Language Models (LLMs) are fundamentally changing the way we gather and analyze data, unlocking capabilities that empower companies to make better decisions, develop more effective strategies, and stay ahead of the competition.
But there are critical questions that arise with the rapid expansion of this game-changing technology. How should it be used? What are the benefits? What are the risks?
Remesh CEO Gary Ellis and CTO Dan Reich shed light on how Remesh is using LLMs, and what researchers need to know as they navigate this ever-changing landscape.

Generative AI and Large Language Models (LLMs): What are these technologies and how are they related?
Dan: Generative AI is a type of Artificial Intelligence (AI) that can generate new and original content, such as images, music, and text.
Large Language Models (LLMs) are a type of Generative AI model that are trained on huge amounts of data — typically text — to generate extremely coherent language. They can be used for natural language processing, machine translation, text generation, and a variety of other language tasks. Some of the more advanced models, like GPT-4, are multimodal, meaning they can take in text and images and output text.
How is Remesh using LLMs?
Gary: Remesh remains committed to being the best-in-class tool for empowering researchers to collect and process qualitative data easily. As new technology emerges that helps make that process more efficient, Remesh will work as quickly as we responsibly can to integrate it into our offering. At present, Remesh is using LLM technology to aid users in the analysis portion of the research process, such as through automated summarization and classification. While we are currently working on more applications, including the generative side, more R&D is still required to validate its efficacy. For now, we are excited that we can give researchers an even faster first take on what’s in their data, which can drastically shortcut their time to insights and free up their time to dig deeper using their understanding of human behavior.
One of the risks we’re considering around using Generative AI for pure generative work is that there’s a loss of control over the output created. Sometimes Generative AI can “hallucinate” or create false content, or content that is not in line with the intended purpose of the model. Summarization tasks decrease this risk because the bulk of the data being used is provided in the input — there’s a lot less reliance on the training data itself — and therefore better maintains the integrity of the research.
Ultimately, we believe that AI technology like LLMs is best used to help humans — not replace them. Our use of LLMs helps our customers gain game-changing efficiencies that empower them to do their job faster and better, which is so important in today’s fast-paced and demanding world.
Moving forward, Remesh will continue to adopt technologies that empower, not replace, helping democratize insights and transform the research industry while maintaining its integrity.
Why is Remesh using LLMs now?
Gary: Data privacy and data protection concerns are the main reason we avoided LLMs in the past. We’re using the technology now because we can actually guarantee that our clients retain ownership of their data. On March 1, 2023, OpenAI altered their Terms of Service removing their requirement to keep and use their customers’ input data. That change made us feel confident we could incorporate LLM technology while maintaining our obligations to our customers and their data.
What makes Remesh’s use of LLM technology distinct?
Dan: Summarize (Beta) is our first use of LLM technology. It works using participant responses to generate a few sentences summarizing each open-ended question from the Remesh Conversation. This Beta feature leverages a GPT-3.5 from OpenAI, the creators of ChatGPT.
What’s distinct about how Remesh uses LLM technology is how we pre-process the data before feeding it to the model. Instead of copying and pasting a bucket of qualitative responses, we are using the underlying data from the participant voting exercises to identify a set of representative responses and use those to generate the summaries.
The power of Remesh has always been our core AI model and the participant exercises. Rather than the researcher needing to independently determine which responses are important or representative, Remesh provides a mechanism for having the respondents themselves identify exactly what is important and what resonates. This extra dimension of data that we provide directly informs the summaries, which means we can provide a true high-level overview to kick-start the rest of your research workflow.
How is this different from how Remesh has used AI in the past?
Dan: The AI and Machine Learning we’ve used in the past (and still use) is leveraged primarily for interpretive and analytical tasks. For example, we use responses and extract relative sentiment, or combine those responses with the voting exercises and compute percent agreement. These tasks and many of the others we use in our platform generate non-unique outputs, typically that require additional interpretation. Multiple responses can generate the same output value from sentiment analysis, typically a numeric value which needs to be processed to generate a coherent output (“positive,” “negative,” “neutral,” etc.).
Summarize, on the other hand, is used for generative work. The system takes responses and generates a summary that represents a unique and creative expression — this output text theoretically did not exist anywhere prior to its generation. Further, these outputs typically need no additional interpretation — they are coherent, human-like sentences.
But despite this, it’s still important to keep actual human researchers in the mix.
Will AI replace the need for humans in research?
Gary: No, AI will help humans achieve greater efficiencies, but it won’t replace them; at least not for the foreseeable future.
AI has a risk of inaccuracies and missing the key insight or application of that insight, and therefore the need for human oversight will remain. The opportunity lies in the increased efficiency of the “right” output that results from combining human expertise with the capabilities of AI and other powerful technologies.
Researchers are uniquely positioned to benefit from the increased capacity provided by the efficiency these new tools offer. This will enable them to dig deeper into the data, spend more time on outcomes and carry those outputs — including the ever-important why — further into and up into organizations.
For example, it can take researchers dozens of hours to sift through open-ended responses manually. With Summarize, that time can be drastically cut. While human oversight is still required to craft the insights and story that the findings reveal, the time savings allows researchers to spend more time on that story, resulting in better outcomes.
What are potential pitfalls tech companies and researchers need to consider when using generative AI technology?
Gary: This is a big question, and I see two key potential pitfalls: blind trust and biased inputs.
- Blind trust
The biggest temptation will be to have blind trust in the technology — to take the LLM outputs “as is” and accept them without question. This can lead to worse business outcomes.
Industry leaders that achieve the best business outcomes will use Generative AI responsibly, meaning they’ll use the results of a generative model as a starting point, not the finish line. We’ve heard from our customers using our Summarize feature that it’s important for them to see the underlying data that comprise the summaries. This transparency is key. Summaries are not insights. While Summarize provides researchers a head start, they still need the raw data to derive understanding and value. Complete transparency allows researchers to leverage their expertise with greater effectiveness.
- Biased inputs that go unchecked
While LLMs can generate highly coherent text, they don’t actually have any understanding of language. Their output is only as valuable as their inputs and their training data, and they can be biased if the data they are trained on isn’t objective. Given that they are trained on data from the web that is inherently biased, these models are inherently biased too.
When unchecked, this can be a big problem. Even without bad intention, biases and stereotypes could be reinforced. Misinformation can be presented as fact, and the effects of that can be far-reaching. This is why transparency and human oversight is so important. Ultimately, there’s a shared responsibility between research professionals and technology partners to remain informed of the potential bias in the models and put measures in place to both identify and address them. This includes complete transparency on how the AI model works.
Data privacy and security concerns: How do we address these and who is responsible for addressing them?
Dan: Data privacy, security, and ownership are huge concerns with the rapid innovation of AI technology because it relies on massive amounts of data. At Remesh, we take this very seriously, which is why we haven’t adopted LLMs until the recent removal of the requirement to keep and use customers’ input data.
Gary: Moving forward, data concerns related to AI technologies is a larger discussion that needs to involve more than just companies like Remesh. We’re leading these important conversations, but they also need to include regulatory bodies and ultimately, society as a whole. We have to decide as a society where the limits are and what guardrails are necessary for responsible use? Because in the long run, technology itself is only as limited as we decide to make it.
Given the risks and limitations, why should organizations and researchers lean into this technology now?
Gary: We’re living in a time of economic uncertainty, budget cuts, and shrinking timelines. The natural tendency during times like this is to cut corners and stop conducting research. This innovation changes that, because in a way it cuts corners and gets organizations speed to insights that can give them more certainty as they move forward.
Now more than ever, researchers and brands need to listen to and understand consumer and employee needs — and they need to get to actionable insights fast. The world is moving at lightning speed, and organizations that don’t keep up risk being left behind.
As a technology partner, Remesh will continue to lean into AI technology and empower our customers to leverage it in a way that is ethical, safe, and hugely beneficial. We will continue to be open and transparent about how it is being used, and create an environment of continual feedback and open conversation as we grow together.
Does it need to be disclosed when outputs contain generative AI?
Gary: Yes, research professionals have an ethical responsibility to disclose when the outputs, in part, contain Generative AI. It still needs to be determined who owns the outputs generated by AI. In the US, images generated by AI were deemed not to be copyrightable. It could take years before the ownership of output discussion is settled. Until then, researchers will want to opt for disclosure to meet their clients' regulatory requirements and maintain trust. As a technology provider, we aim to empower the researcher to uphold their client's ethical standards; and we will always be forthright and transparent about when we use Generative AI and its capabilities. Our internal ethics committee regularly monitors changes and implications to determine the best path forward.
What’s next: How will LLMs help shape the insights industry?
Dan: LLMs hold a substantial potential to revolutionize the insights industry. In the short term, they are likely to serve an integral role in getting from data to output to insights. We already see them being used for text summarization, theme extraction, and some high-level data analysis. But they are likely to move to other tasks as well that span the research process.
In the not-too-distant future, I expect to see LLMs involved in creating and initiating research projects. We will likely see LLMs helping to write proposals, write the actual research plans, and even recommend participant segments. Further, it wouldn’t surprise me to see LLMs actually running research altogether via some sort of chat interface or even using text-to-speech.
We will inevitably see this technology incorporated everywhere throughout the industry — it’s not going anywhere. However, we should always keep in mind that this innovation brings both tremendous potential and significant responsibility. As we continue to develop and refine these technologies, the ethical considerations surrounding their use remain paramount. So, as we forge ahead, we must do so with a steadfast commitment to maintaining the highest ethical standards.
Ready to see Remesh in action? Reach out to our team and will connect you with a dedicated product expert.
-
Lorem ipsum dolor sit amet, consectetur adipiscing elit. Suspendisse varius enim in eros elementum tristique. Duis cursus, mi quis viverra ornare, eros dolor interdum nulla, ut commodo diam libero vitae erat. Aenean faucibus nibh et justo cursus id rutrum lorem imperdiet. Nunc ut sem vitae risus tristique posuere.
-
Lorem ipsum dolor sit amet, consectetur adipiscing elit. Suspendisse varius enim in eros elementum tristique. Duis cursus, mi quis viverra ornare, eros dolor interdum nulla, ut commodo diam libero vitae erat. Aenean faucibus nibh et justo cursus id rutrum lorem imperdiet. Nunc ut sem vitae risus tristique posuere.
-
More
.jpg)
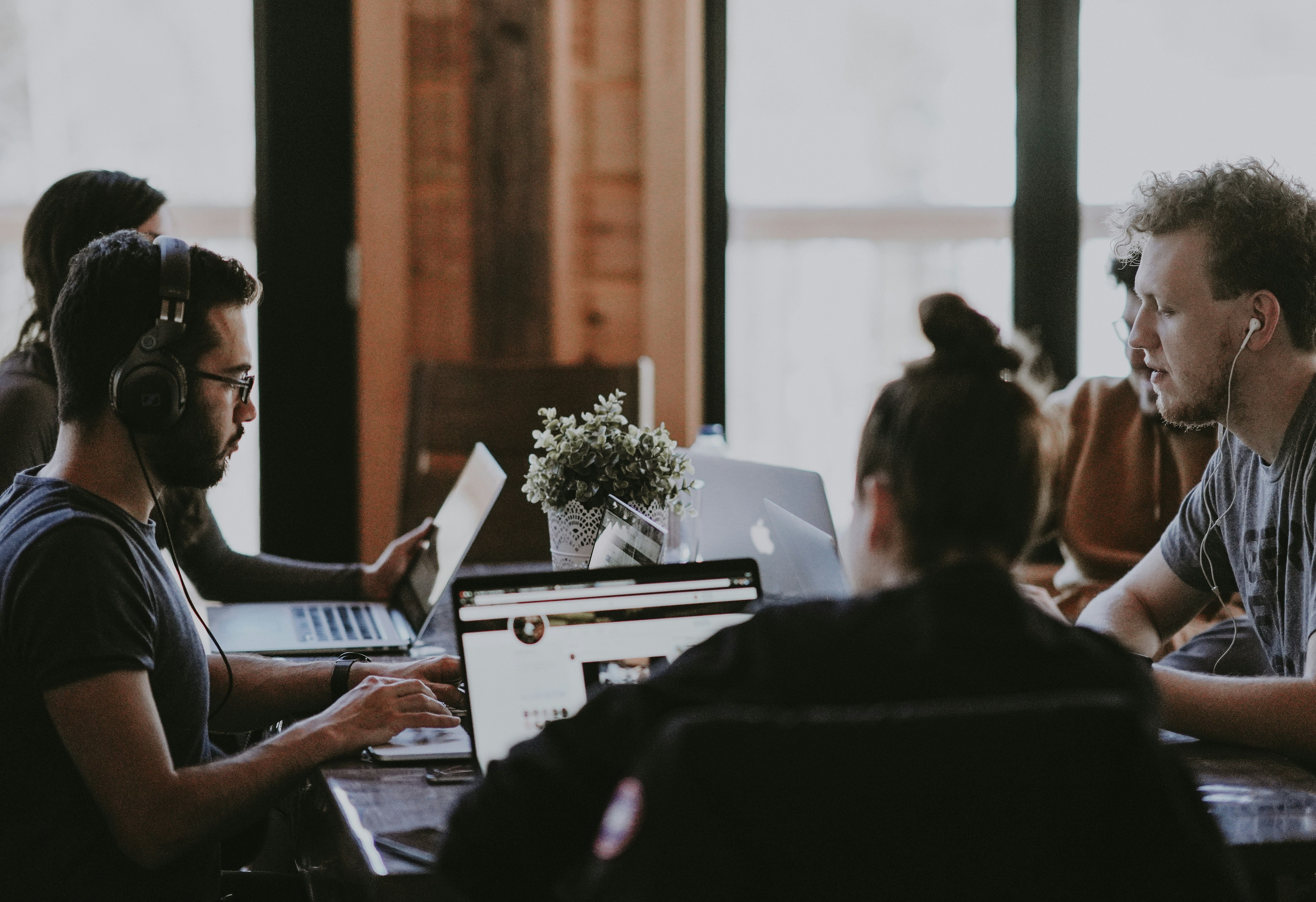

Stay up-to date.
Stay ahead of the curve. Get it all. Or get what suits you. Our 101 material is great if you’re used to working with an agency. Are you a seasoned pro? Sign up to receive just our advanced materials.