
Advanced Research
Taking a Dialogic Approach to Change
Patrick Hyland
January 10, 2025

Advanced Research
Taking a Dialogic Approach to Change
Patrick Hyland
January 10, 2025
.jpg)
Advanced Research
Creating a Culture of Belonging: Insights Every Leader Should Know
Patrick Hyland
January 10, 2025
Articles
.jpg)
Advanced Research
Creating a Culture of Belonging: Insights Every Leader Should Know
Patrick Hyland
January 10, 2025
Articles

Research 101
Ideation Tool: How Remesh Transforms Idea Collection and Testing
Team Remesh
November 12, 2024
Market Research
Articles

Research 101
Ideation Tool: How Remesh Transforms Idea Collection and Testing
Team Remesh
November 12, 2024
Market Research
Articles

Advanced Research
How Gen Z Leadership Expectations Are Shaking Up the C-Suite
Anthony Caputo
November 4, 2024
Articles

Advanced Research
How Gen Z Leadership Expectations Are Shaking Up the C-Suite
Anthony Caputo
November 4, 2024
Articles

Advanced Research
Qualitative Research Methods: When Medium Matters (And When It Doesn't)
Team Remesh
November 4, 2024
Articles

Advanced Research
Qualitative Research Methods: When Medium Matters (And When It Doesn't)
Team Remesh
November 4, 2024
Articles
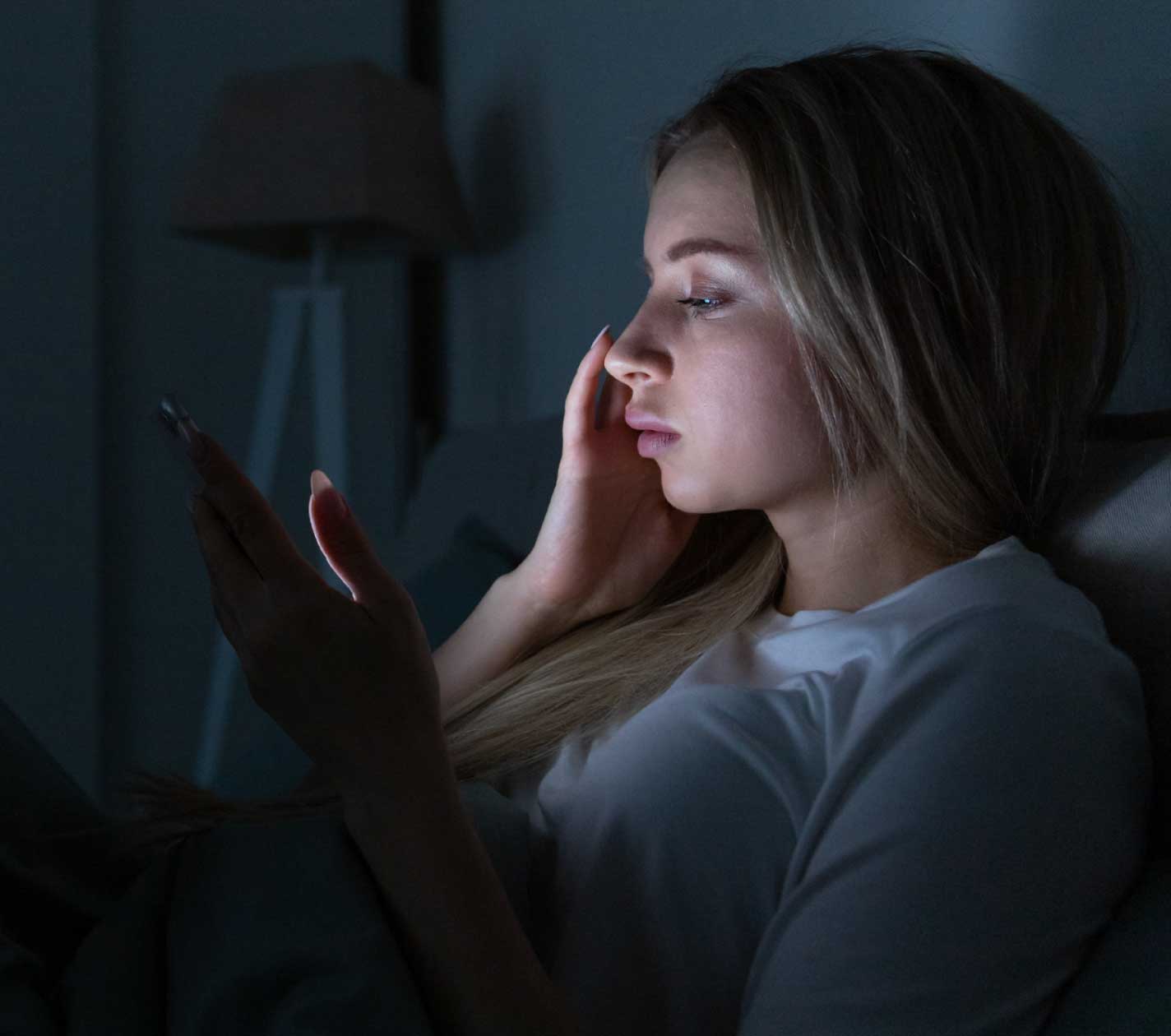
Advanced Research
Mastering Rapid Shifts in Consumer Behavior in Real Time
Team Remesh
November 4, 2024
Articles
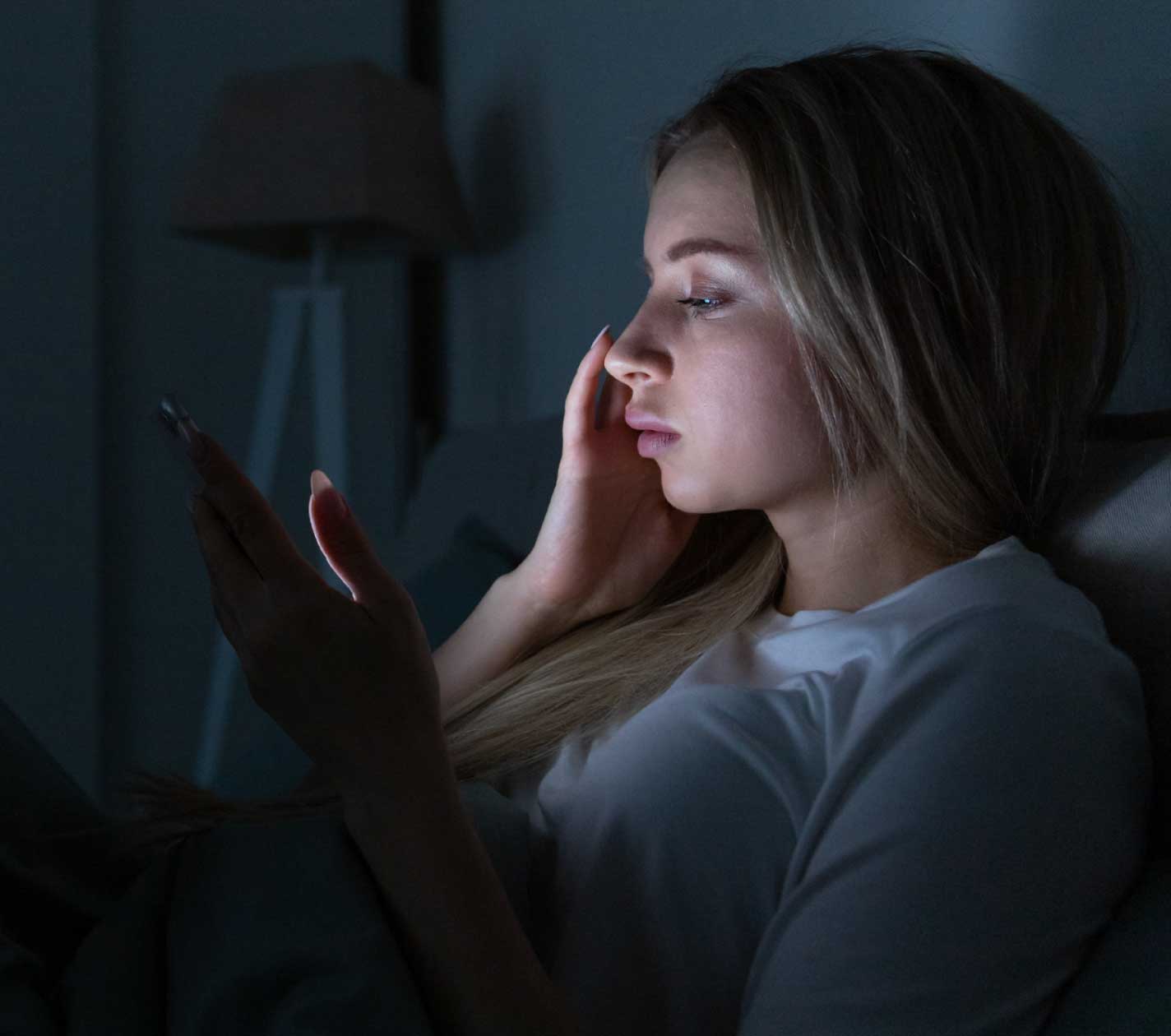
Advanced Research
Mastering Rapid Shifts in Consumer Behavior in Real Time
Team Remesh
November 4, 2024
Articles
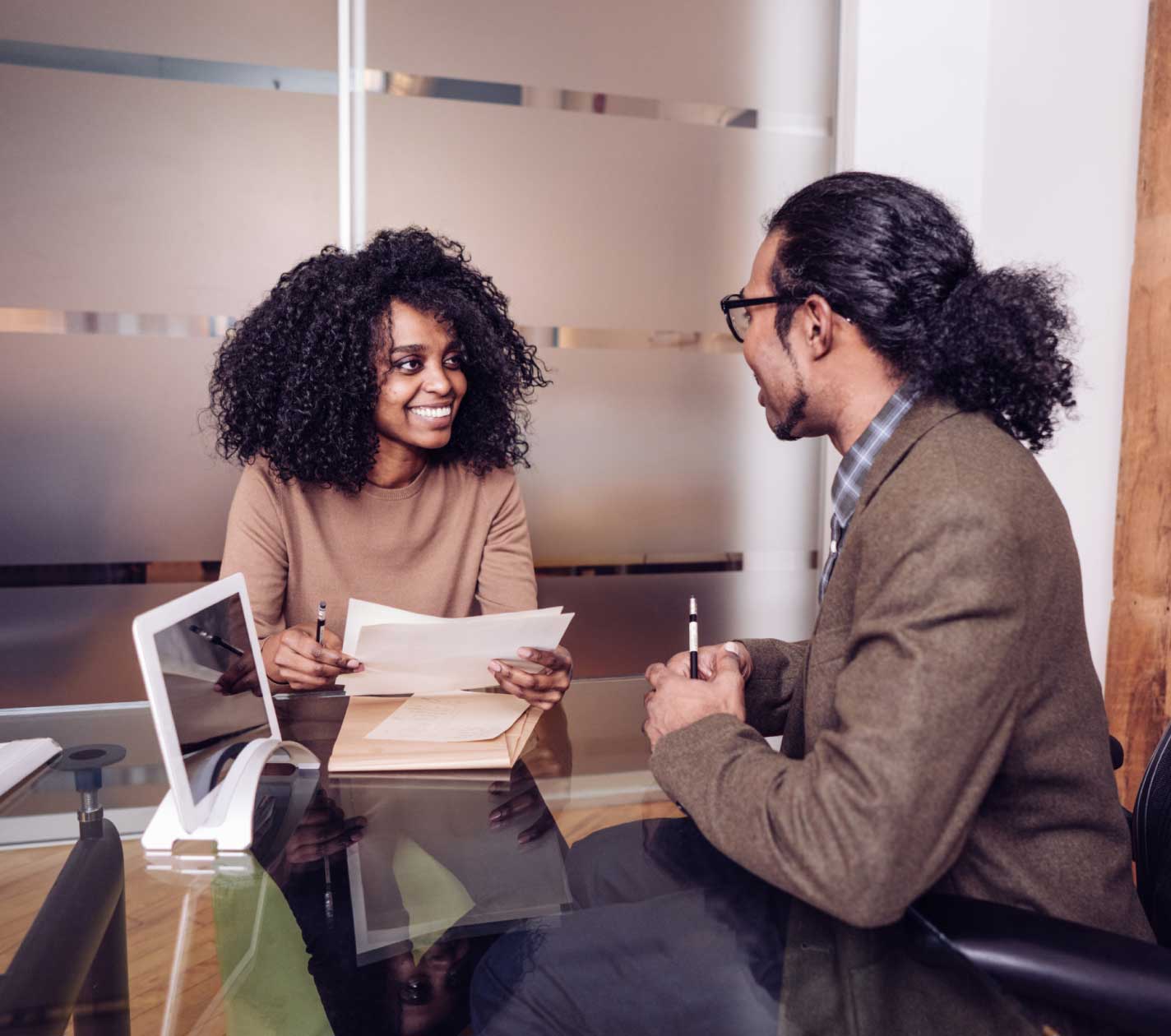
Advanced Research
How Narrative Research is Transforming Consumer Insights
Team Remesh
November 4, 2024
Articles
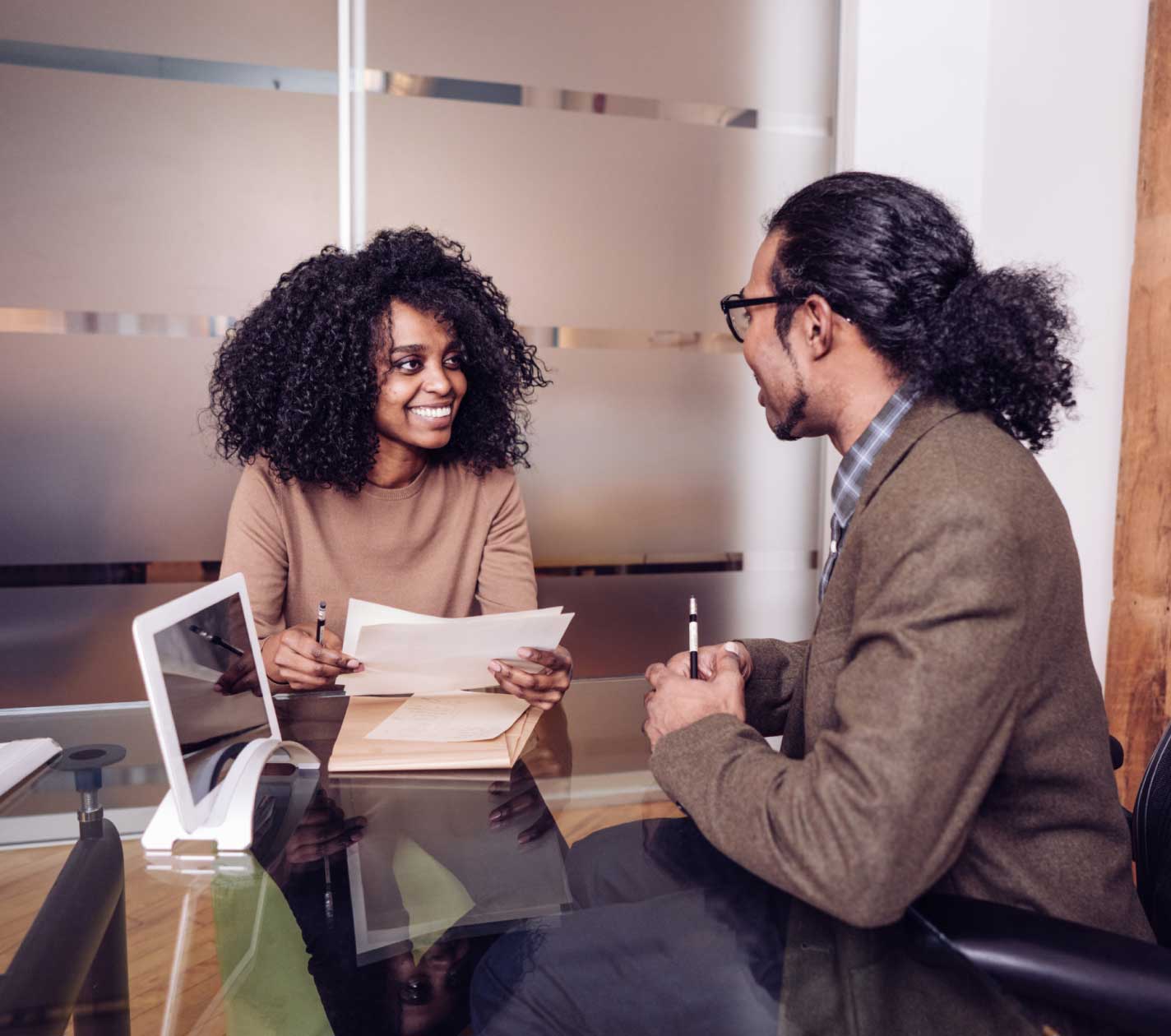
Advanced Research
How Narrative Research is Transforming Consumer Insights
Team Remesh
November 4, 2024
Articles
The Evolution of AI in Market Research: My Perspective
AI is rapidly changing how market research is conducted, from predictive modeling to large language models that can analyze vast amounts of data in seconds. This article explores AI's impact on research workflows and emerging capabilities, while examining the vital role human expertise still plays in this evolving field.
.jpg)
AI in market research has come a long way already, and it’s just getting started.
To understand this evolution, we first need to understand the broader evolution of AI itself. So, let’s take a moment to reflect on where AI in market research has been and where it’s going.
Even before the predictive modeling breakthroughs of 2014, AI was no newcomer. As far back as the 1980s, "expert systems" represented a major AI innovation. These knowledge-focused programs helped corporations automate complex tasks in data mining, robotics, logistics, speech, and medicine.
The 90s saw AI continue major strides across machine learning, tutoring systems, reasoning approaches, and language comprehension. So, the building blocks were being put in place for AI's eventual application to market research. While limited compared to today's capabilities, these earlier eras of AI progress set the stage for the transformative predictive modeling and natural language advances that kicked the AI and market research relationship into high gear starting in 2014.
Early predictive models
Things really kicked up around 2014 when we saw the emergence of AI technology that could make predictions - even from fuzzy inputs. This enabled the initial application of AI to market research - using predictive models to forecast insights from limited survey responses.
For example, we could ask an open-ended question to 1,000 people, get 1,000 textual responses, and then have only 10-20 people vote on those responses to predict how the broader group would vote. This hugely scaled up our ability to analyze open-ended, conversational data.
A few years after the initial predictive modeling applications, breakthroughs emerged in natural language processing that enabled new large-scale innovations in areas like text analytics.
These mid-2010s innovations marked a meaningful step forward.
The birth of Remesh
That’s also when AI-powered Remesh was born.
Back when Chief Science Officer, Andrew Konya, was a physics grad student, he made friends with folks on opposite sides of a conflict. When they argued, he saw leaders rarely spoke for most people. This led to an important question - could tech give groups a collective voice?
This idea drove Andrew to a hackathon. He aimed to create something to understand what everyday people think. They had 48 hours to build it - minus the two or three hours of sleep.
They worked hard through the nights, fueled by caffeine. By mashing up code, they built the first version of our software. It could instantly gather regular people's views on issues. Remesh was born from a dream to grasp the views of the different sides better.
It was called Remesh - a name that worked well enough that first night.
Soon, Andrew realized what began at a hackathon now needed to grow into a real product. Today, Remesh proudly serves major interests in non-profits, the public sector, small companies, and large enterprises.
The Next Big Leap: Large Language Models
But all of that was just a prelude to the huge leap forward with large language models (LLMs) like ChatGPT.
The scale of data and computing that these models can leverage results in remarkably general capabilities that mirror human abilities. With the right prompts, LLMs can process language, reason about knowledge, summarize texts, answer questions, generate content, and even hold conversations, as well as most humans can.
The emergence of LLMs has had a bigger impact on market research than everything that came before combined. After all, its capabilities align so well with core market research capabilities of making sense of qualitative data and eliciting insights from consumers.
Tasks that once took trained researchers hours or days can now be accomplished in seconds.
For example, LLMs can instantly summarize consumer feedback into succinct key takeaways that can be emailed to stakeholders. They can answer natural language questions about datasets as if they have read and deeply understood the data. They can even generate entire sets of survey questions or guides to interact with consumers, needing only high-level guidance like a research objective as input.
So, in a very short time, LLMs have massively expanded what is possible with AI in market research. All in all, half of businesses are using artificial intelligence in at least one business function.
And we're still just scratching the surface.
Adoption Trends in Market Research
LLMs spread like wildfire in the market research industry because their core capabilities aligned so well with researcher needs. Plus, models like GPT-3 were easily accessible to anyone through user-friendly interfaces. At the time, you could go to one industry conference where AI was barely mentioned one quarter, then completely dominated vendor messaging the next quarter after GPT-3 launched.
Researchers are finding meaningful applications among their standard workflows, including using AI to:
- Draft research questions
- Analyze volumes of responses
- Output usable, actionable insights
- Summarize key themes
- Model customer sentiment over time
- Write initial draft reports
- Create dialogue at scale
And more…
And leading-edge teams are inventing entirely new rapid-cycle conversational approaches powered by AI.
So, adoption has been exceptionally quick already, but we're still just getting started. I think the general arc that we will see follows this progression:
- The task is done by humans
- Then, it's done by humans with AI help
- At some point, it’s done by AI with humans overseeing it
- Fully done by AI
The key for researchers is to ensure they find the right use cases where AI can help them the most.
Developing a Strategic Approach
For research teams getting started with AI today, the key is developing a strategic approach tailored to your strengths. Here are a few best practices I recommend:
Identify the most tedious, manual, and error-prone parts of your workflow that could benefit from automation. Applying AI here will allow researchers to use their time more productively.
Ensure staff builds direct experience by asking, probing, and even stumping the AI. Determine what works and what doesn’t while keeping your needs in mind.
Use AI to optimize your workflows and keep up with the speed of business.
Experiment with use cases over time continuously. Technology evolves rapidly, so keeping up requires dedicated learning efforts.
Researchers who invest in expertise and use of the right AI capabilities will have access to market research workflows and speeds unlike anything previously possible.
The Role of Humans in AI-Assisted Market Research
While visions of fully automated market research processes may dance through some minds, I think human judgment, oversight, and course correction will remain essential in five key areas for the foreseeable future:
1. Setting Overall Research Direction
Humans must determine core objectives, populations of interest, and research needs to frame projects. AI can subsequently help design research instruments and interact with samples to fulfill human-defined goals.
2. Validating AI Outputs
AI-generated insights, questionnaires, conversational flows, and analyses should all be reviewed by experienced researchers - even if it is just spot-checking.
3. Interpreting Results
Once research outputs have been validated for accuracy, making sense of the meaning of situations at hand depends on human judgment to account for nuances in context AI may miss.
4. Recommending Strategic Decisions
The human researcher should still validate and evolve the strategic decisions the AI output seems to suggest based on their experience and understanding of the project.
So, while AI promises to expand what is humanly possible in market research, humans themselves are not getting replaced completely. The most valuable result of the collaboration is letting AI greatly boost research volume, scale, and processing speed while humans provide oversight for quality, meaning, and wisdom.
The Future of AI in Market Research
AI-human collaboration in market research is rapidly becoming integral. We can expect this hybrid model combining both strengths to become increasingly standard practice.
The march of technological progress is opening new fronts in applying market research at scale. AI promises to transform market research.
Here are two cutting-edge capabilities starting to emerge that hint at a radically different future:
AI Dialogue at scale
AI's natural language capabilities can collect perspectives through group conversations at scale. Rather than individual surveys or interviews, language models can engage whole segments in collaborative dialogues to gain insights.
For example, AI can facilitate dialogues at scale around product concepts, adapting in real-time based on reactions and drilling into areas of interest.
These collective conversations around topics driven by AI promise dynamic insights compared to periodic surveys or polls. Language models can capture evolving themes from organic dialogues among populations of interest.
Synthetic Respondent Panels
Today, capturing insights from large, diverse consumer samples requires costly recruiting and interview processes. However, trained AI models can mimic consumer segments.
As confidence quantification gets solved, market research without paid human respondents may become possible through what are essentially AI simulations.
Final thoughts
The era of AI-powered market research has clearly arrived, but the best AI for market research use case depends on your specific requirements and pain points.
Are inefficient workflows slowing your team down? Intelligent automation may help. Do volumes of qualitative data overwhelm? Summarization and visualization tools assist. Do you need to move faster? AI can help.
AI greatly expands what is humanly possible, allowing market research at scale once unthinkable. Meanwhile, human oversight ensures quality control and contextual wisdom machines lack.
The power comes in aligning the right AI tools to the right use cases for each researcher.
-
Lorem ipsum dolor sit amet, consectetur adipiscing elit. Suspendisse varius enim in eros elementum tristique. Duis cursus, mi quis viverra ornare, eros dolor interdum nulla, ut commodo diam libero vitae erat. Aenean faucibus nibh et justo cursus id rutrum lorem imperdiet. Nunc ut sem vitae risus tristique posuere.
-
Lorem ipsum dolor sit amet, consectetur adipiscing elit. Suspendisse varius enim in eros elementum tristique. Duis cursus, mi quis viverra ornare, eros dolor interdum nulla, ut commodo diam libero vitae erat. Aenean faucibus nibh et justo cursus id rutrum lorem imperdiet. Nunc ut sem vitae risus tristique posuere.
-
More

.jpg)

Stay up-to date.
Stay ahead of the curve. Get it all. Or get what suits you. Our 101 material is great if you’re used to working with an agency. Are you a seasoned pro? Sign up to receive just our advanced materials.